Translation
Author: Xingxing Cheng
In 2022, with numerous autonomous driving companies entering the intelligent driving track, L2+ navigation assisted driving products are evolving and maturing at a visible speed. However, upon closer inspection, this evolution and maturity is still limited to high-speed scenarios, and there are almost no navigation assistance products that can meet the stronger demands of urban scenarios for the working class.
But this deadlock will be broken in mid-2023, and the one who will break this deadlock is the powerful player in autonomous driving – Baidu. On November 29, 2022, at its technical event – Apollo Day, Baidu launched a flagship product ANP3.0, an L2+ navigation assisted driving system that can support complex urban road scenes in China, and integrates seamlessly with high-speed and parking scenes.
Three weeks later, on December 16, Baidu released a video of ANP3.0’s generalized road test. The test vehicle not only appeared on typical and characteristic Chinese roads such as Yuanmingyuan West Road, Hu-Jia Expressway, Dongbin Tunnel, but also was able to cope with various work scenarios such as ETC (Electronic Toll Collection), temporary traffic signals, and close-range cutting in traffic with ease, making me excited and enthusiastic.
The ability of three-domain integration is the highest martial arts skill pursued by every company in the industry, but unfortunately, there are few who have successfully mastered it. Facing such complex urban road conditions, ANP3.0, which is still in the generalized testing stage, has already mastered this skill solely relies on pure visual perception. Once the configuration of the dual-redundancy plan with laser radar is added in the mass production stage, its experience will undoubtedly bring surprises to the industry and will also be a big event in the industry.
In the following section, we will start with the skills showcased in the generalized road test video and gradually unearth the profound skills that Baidu Apollo learned.
Every move showcases the hero’s true colors
Linking the ramp and toll station connects the urban and high-speed scenarios, which is a typical scenario that is easy to defend and difficult to attack, and a must-fight battlefield for navigation assistance driving products.
Toll station scenarios are widely recognized as having a high degree of difficulty. The spacious toll plaza without lane lines, the ETC toll sign constantly switching under the toll station canopy, the narrow toll island channel, the vehicles in front that stop and go, and the thin and panic-inducing toll pole all challenge the vehicle’s perception, planning, and control to the utmost.
Therefore, most of the road test videos of navigation assisted driving products from various companies generally require human intervention when approaching toll stations. However, in order to connect high-speed and urban scenarios, ANP3.0 has achieved the unique automatic ETC pass-through function in the industry. From the road test video, it can be seen that ANP3.0 can intelligently choose the optimal ETC lane based on its position when entering the toll plaza, decelerate before entering the toll island channel to facilitate better ETC recognition, and accelerate through after recognizing the toll pole rising.
This move should be like the famous swordplay of Dugu Jiujian, “Breaking Sword Style”, where I have something others do not.
After entering the heart of the city, the number of traffic participants on the road suddenly increases, including the elderly, children, cats, dogs, bicycles, and e-bikes. **In the urban scene, unprotected left turns are one of the most difficult situations. Understanding and conquering this situation is equivalent to advancing to the finals of intelligent driving in advance. **
Unprotected left turn refers to a crossroads without a dedicated left-turn signal and shares the same traffic light with straight traffic. In the case of an unprotected left turn, the vehicle needs to follow traffic rules, judge whether the vehicle can turn left based on the traffic signal, and at the same time, try their best to yield to oncoming vehicles. On the other hand, it needs to have game strategies, pass on the intention to turn left to oncoming vehicles through gradual slowing down and stopping, and accelerate through the straight lane after judging that there is a safe distance from the straight-moving vehicles. After accelerating through the straight lane, it needs to immediately slow down and perceive and predict pedestrians on the zebra crossing and non-motorized vehicles on the bicycle lane in real-time, so as to safely and efficiently enter the opposite lane.
There are two difficulties in unprotected left turn. One is that the perception of the vehicle itself cannot reach beyond the visual range, so it cannot obtain information about occluded oncoming vehicles, non-motorized vehicles on the bicycle lane, and the movement of pedestrians on the zebra crossing in advance. The second is that it cannot incorporate the understanding of human intentions into decision-making. Without beyond visual range perception, it is impossible to achieve overall planning and control in a smooth and coherent manner.
In the road test video, ANP3.0 showed us its ability to play games in a busy intersection. At first, the dense straight traffic made the test vehicle unable to move at all. I believe even experienced drivers would encounter similar situations. However, after the straight traffic became slightly sparser, the test vehicle adopted a strategy of gradual approaching and eventually successfully made a left turn after recognizing a safe distance.
There are also similar situations of game-like challenges in the urban scene, such as unprotected U-turns, mixed non-motorized vehicles and motor vehicles at intersections, which were also demonstrated in the ANP3.0 road test video. Playing games is currently a challenge for the industry since it is easy to judge the distance and speed of approaching vehicles through the sensors on the vehicle, but it is difficult to understand the intentions of other drivers or pedestrians through a glance or a wave.These moves represent game theory, and are no doubt the “Tai Chi” of softness over hardness.
Whether in urban or highway scenes, the handling ability of the most common work condition determines the lower limit of user experience. That’s why, some days ago, Xinchuxing had to conduct comprehensive tests on the LCC capabilities of this year’s mainstream smart electric cars, and the results were disappointing. Cutting cars at close range either failed to recognize the preceding car in time, or caused a dizzying brake.
Let’s discuss how ANP3.0 performs in these basic abilities and whether it can meet people’s daily travel needs.
(1) Traffic light response capability. Traffic lights are important devices that reflect traffic rules in urban scenes. Incorrect perception can disrupt traffic, or even cause accidents. The changing shapes of traffic lights demand high vehicle perception abilities. The ANP3.0 road test video shows a crossing test resembling a temporary traffic light intersection, which is very challenging.
(2) Close-range cutting car response. Cutting cars at close range is one of the most common work conditions in urban scenes. Due to the close distance, it is easy to miss perception, which can lead to collisions. From the ANP3.0 road test video, we can see that when the tested vehicle behind shows an intention of cutting in, the system has already started response strategies. After the cutting car passes the lane line, the system begins to smoothly decelerate and avoid the car, like an experienced driver.
(3) Non-motorized mixed traffic response at intersections. Non-motorized mixed traffic is a difficult problem in the industry, especially with unexpected conditions. Most products either cannot recognize the hazard or react too late. The ANP3.0 system demonstrated in the road test video that it can predict and track non-motorized vehicles on both sides of the intersection. Once the system predicts the intersection will appear in the future, the vehicle will smoothly decelerate and avoid the intersection with human care.
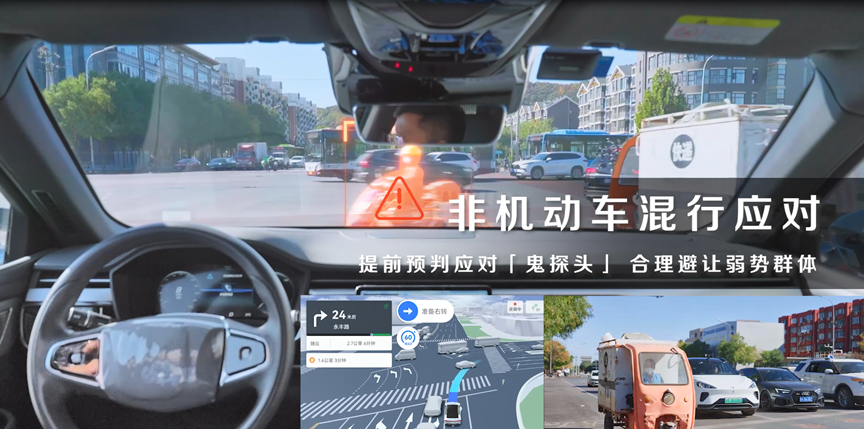
Except for the typical and challenging working conditions mentioned above, ANP3.0 also shows the smoothness of an experienced driver in handling obstacle avoidance, intelligent avoidance of large vehicles, tunnel crossing, and extreme curve driving.
The move that reflects basic skills would probably be the "horse stance," which is a must-learn for those with excellent skills.
The few minutes of video only show a small part of ANP3.0's capabilities. During the 119KM road test from the Baidu Building car park to the Badaling Great Wall, ANP3.0 was autonomous for the entire journey, without any human intervention. The journey included passing 27 traffic lights, 2 ETC stations, 6 left turns, 1 unprotected U-turn, 1 ramp, 10 tunnels, 20km of mountainous roads, 9 obstacle avoidance maneuvers, 6 yield-to-pedestrian situations, 19 sudden braking situations, 8 large vehicle avoidance situations, and 17 self-initiated lane changes.
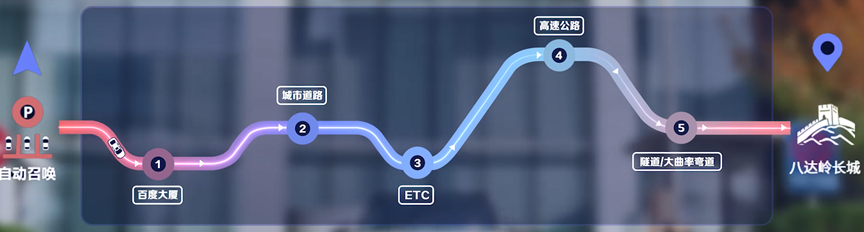
It seems that ANP3.0 is well-prepared for this challenge and aims to become the leader in this field.
## Every little bit is built on a solid foundation
We can't help but ask, what kind of internal strength and technique is needed to achieve such gorgeous and solid moves? We can find the answer from the Apollo Day on November 29th.
### The first layer of internal strength: Practical hardware architecture
I have always believed that blindly adding hardware components is a sign of insufficient internal strength. The key to success lies in the practical hardware architecture. For the calculation unit of ANP3.0, it uses a self-developed intelligent driving domain controller, powered by two widely used Orin X chips, with a computing power of 508Tops. This hardware configuration is considered the standard in this year's market of intelligent electric vehicles. The domain controller is equipped with a water cooling system that can maintain a temperature range of -40°C to 85°C, which shows excellent engineering capabilities.
In terms of sensors, during the testing and development phases, pure visual perception was used, including seven high-definition cameras with a visual range of 400 meters and 8 million pixels, as well as four 3-million-pixel high-sensitivity omni-directional cameras, showing very good results on the prototype road test. In the production phase, laser radar will be added to form a safety double redundancy with vision. The location, number, and specifications of the laser radar can be flexibly adjusted based on the needs of our partners.
The second level of internal skills: the redundant visual + Lidar dual-sensing solution in the industry.
ANP3.0 adopts Apollo’s characteristic “BEV surround four-dimensional perception”. During the development and testing stage, the perception solution was completely visual. Even the recently released video of 119 km of road testing was based on a pure visual perception solution. This makes ANP3.0 the only pure visual automatic driving perception solution in the world and the only one in China that can support complex urban roads.
However, in the mass production stage, based on an understanding of actual corner cases, ANP3.0 will use Lidar as a redundant perception system to better cope with unconventional and night obstacles, dynamic/static occlusion detection, and complex urban scenarios such as multi-storey parking lots.
The ANP3.0 visual and Lidar sensing systems operate independently with low coupling, making it probably the only true redundant environmental perception solution in China.
The visual perception technology behind ANP3.0 has also been developed through several years of practice. In 2019, Baidu launched the Apollo Lite project, which adopted the technology framework of “monocular perception” plus “surrounding fusion”. Based on deep learning technology, the project includes 2D target detection and 3D pose estimation. After single-camera tracking, multi-camera fusion, and other steps in the back-end processing, the final perception results are output.
At first, Apollo Lite performed satisfactorily, but as the testing scale gradually expanded, it gradually became inadequate in solving corner cases that required multi-camera collaboration. At the same time, rule-based tracking and fusion solutions failed to fully leverage data advantages, becoming a bottleneck in rapidly improving perception capabilities.
For this reason, Baidu upgraded the visual perception technology framework and released the second-generation pure visual perception system, Apollo Lite++. Based on the transformer, Apollo Lite++ converts the front-facing feature to BEV and directly outputs four-dimensional perception results after front-end fusion of camera observation at the feature level.
It is precisely based on the evolution of Apollo Lite++, that ANP3.0 has become the only one in China that can run through urban domains and multiple scenes relying on pure visual perception solutions during the development and testing stage.
With BEV surround four-dimensional perception, is the perception system impeccable? The answer is not necessarily. Imagine a scenario where thick trees obstruct traffic signs. At this time, neither the camera nor the Lidar can accurately recognize the sign, leading to either violating traffic regulations or causing traffic accidents.
The third layer of internal skill is high-precision maps that can solve beyond-line-of-sight perception.
There is a growing consensus in the industry that high-precision maps are necessary for safe and high-quality navigation-assisted driving products. Leveraging its rich experience in mapping, Baidu has explored a low-cost, high-freshness approach to high-precision map production.
1. Cost reduction and efficiency improvement in the collection phase
One of the main features of high-precision maps is their high accuracy, which needs to be at the centimeter level. In order to improve accuracy and reduce errors, map makers usually collect data from the same route multiple times, which makes the collection phase inefficient and costly. However, by relying on years of experience in mapping and deep algorithmic expertise, Baidu has innovatively improved the tolerance of map accuracy errors by optimizing the online PNC algorithm and upgrading the map point cloud stitching algorithm. This way, each route only needs to be collected once, significantly reducing the collection cost and increasing efficiency.
2. Automation of data fusion
The goal of data fusion is to integrate sensor data collected multiple times into a unified coordinate system, which is an important foundation and core capability for large-scale production of high-precision maps. The challenge is to achieve centimeter-level accuracy in the fusion process. The conventional approach is to divide the sensor data according to blocks or roads and then fuse the data in each block sequentially.
Baidu’s approach is to construct a multi-level graph structure by dividing the data space, ensuring that the accuracy of the entire map is consistent.
3. Dimension reduction of the positioning layer
In a hierarchical high-precision map structure, it was previously necessary to produce and store three layers of feature points, laser point clouds, and landmarks, which added pressure to the production process and online storage. However, Baidu Apollo can support ANP3.0 urban road high-precision self-positioning with only the landmark positioning layer by upgrading the positioning algorithm and reducing reliance on point clouds and feature points.
4. Streamlining the labeling of map elements
High-precision maps not only contain many physical world road elements but also require complex associated relationships to be labeled for automated driving algorithms. For example, in the case of intersection traffic light recognition, each light head previously required separate labeling, and the light heads also needed to be bound to their corresponding lane lines, which was a tedious and error-prone operation for labeling personnel.And Baidu Apollo, by improving its ability to perceive red green lights as well as enhancing its understanding of scene semantics, requires only the annotation of a single box and the establishment of a binding relationship with the stop line for its streamlined annotation, reducing the probability and cost of human error.
The fourth level of internal practice is: data-driven improvement with a continuously flowing source of experience.
At this stage, no one can guarantee that their own products can solve all Corner Cases, so it is wise to use data-driven iteration to optimize algorithms and improve the consumer experience. Leveraging Baidu’s accumulations in AI, cloud computing and autonomous driving, ANP3.0 has created an innovative data-closed SaaS product for both internal and external users. By managing the full lifecycle of data mining, annotation, model training, and simulation verification, data-driven intelligent driving capabilities can be enhanced.
1. Perception system level, ANP3.0 adopts highly integrated AI systems, and over 90% of perception system modules are designed and implemented with learning, enabling continuous evolution through data-driven perception systems.
2. Data collection level, due to the characteristics of cloud driving, long-tail data most needed by each model task can be selectively online mined. Through dynamic mining and vehicle-cloud interaction technology, targeted, dynamic, low-cost mining of data fragments needed to supplement AI model capabilities can be achieved, enabling the rapid accumulation of data assets.
3. Model stability level, in conjunction with the MLOps engineering culture and process mechanism, Baidu standardizes and encapsulates complex model training steps to make the training process more efficient, model output more stable, and to enable rapid iteration and high-priority problem resolution on a daily and weekly basis.
And this internal practice is not just empty talk, but is verified by more than 40 million kilometers of professional road tests. Every day, a massive amount of L4 LiDAR data is generated, supported by a highly automated training data production line for the BEV obstacle perception model of ApolloL2++, enabling faster construction of the next generation of BEV perception capabilities ahead of competitors.
In conclusion, to borrow a phrase from Fernando, the difference between cars lies in whether they have run 40 million kilometers or have run only 10,000 kilometers but have repeated it 4,000 times.With the entry of high-level players and the anticipation of consumers, the intelligent driving track has ushered in a rare development opportunity. In this process, whoever can first integrate the three domains, whoever can first launch technologically advanced urban navigation assisted driving products, and whoever may become the “superhero” in the minds of billions of consumers.
ANP3.0 relies on steady progress, step-by-step. This gives us reason to believe that the urban navigation assisted driving will bloom like the most beautiful flower this summer, and the integration of the three domains will become the brightest star in the night sky.
This article is a translation by ChatGPT of a Chinese report from 42HOW. If you have any questions about it, please email bd@42how.com.