Author | Zhu Shiyun
Editor | Qiu Kaijun
“After sufficient validation in the future, we will no longer choose between the perception results and map information, and we believe in the real-time perception results,” said Ai Rui, vice president of Haomo Zhihang Technology, to Electric Vehicle Observer.
In 2022, many top players in the intelligent driving industry proposed to “focus on perception and reduce reliance on maps” in urban navigation. However, honest solutions still need to operate within the applicable range of high-precision maps.
Haomo believes that urban navigation can be done without maps.
By 2025, the installation rate of advanced driver assistance systems in China will reach 70%. In 2023, Haomo’s City Navigation On-Highway (NOH) relying solely on ordinary navigation maps will be mass-produced and launched on car models, with an estimated landing in 100 cities in the first half of 2024.
Meanwhile, Zhang Kai, chairman of Haomo Zhihang, said that the intelligent driving system mainly based on perception technology and relying on visual schemes can be deployed on mid- to low-power vehicle platforms, making advanced intelligent driving systems possible for mid-priced cars.
The Haomo City NOH laser radar version on the Wei Pai Mocha, launched in 2022, once competed with Huawei and XPeng for the first launch of urban navigation features. However, it did not materialize in the end. Now that Haomo has set a goal of covering 100 cities by 2024, is it still just a “marketing gimmick”? More importantly, how can urban navigation be done without maps?
Light Maps Before Generalization
Although the urban navigation functions that Huawei and XPeng currently provide to small batches of customers still require high-precision maps, “light maps” are still an industry consensus. Huawei intends to launch a light-mapping solution similar to crowdsourcing in mid-2021, while XPeng Motors said that XPILOT 4.0 will adopt a light mapping scheme after initial closed-loop testing.
The reason for “light mapping” is partly due to the freshness issue of high-precision maps.
Currently, companies such as Amap, NavInfo, and Baidu can provide high-precision maps that cover the entire national highway network, the urban expressway network, and even ordinary urban road sections. However, the freshness of most maps that are updated quarterly is entirely insufficient to meet the needs of urban navigation.
And under the high-precision map policy, currently only six major cities including Beijing, Shanghai, Guangzhou, Shenzhen, Hangzhou, Chongqing have launched pilot applications of intelligent connected vehicles with high-precision maps.
On the other hand, there is a cost issue.
At present, many intelligent driving players including Huawei have Class A or Class B qualification for map surveying and mapping, but the high cost of high-precision map drawing makes people hesitate.
For reference, in 2018, DeepMap, an unmanned driving car high-precision map technology company invested a total of 450 million U.S. dollars for development. In addition, the development costs of enterprises such as MapBox, Carmera, and Civil Maps were also 227.2 million US dollars (in 2017), 20 million US dollars, 17 million respectively.
Such costs obviously contradict the development mode of large-scale landing intelligent driving functions, obtaining a large amount of data to promote system iteration.
Zhang Kai told “Electric Vehicle Observer” that the definition of mid- to high-end priced models ranges from 120,000 to 250,000 yuan. In the plan of the next generation intelligent driving platform, Haomo arranged the adaptation of mid- to low-end priced models, where the cost of about 1,500 yuan can achieve on-street parking, high-speed HWA (LCC class function), and the cost of around 2,000 yuan can achieve high-speed NOH function.
Such costs put higher requirements on the prices of perception and computing hardware.
Airui said that Haomo plans to achieve a certain accuracy of NOH function on a 20-30Tops computing power platform in the future.
Therefore, new ways of using maps are needed.
There are two ways to use maps: one is explicit, building offline maps for real-time calling as prior information for the system’s decision-making. When the perception results and map information do not match, the system needs to follow the pre-set logical rules of choosing who to believe in.
The other is implicit, using ordinary map information as prior input to the model for error correction. Just as humans do not see dead ends when driving, they continue to follow the navigation instructions.“`
“So we hope that the model has this ability, which can avoid either/or. (Implicit) methods are theoretically higher, but more difficult.” Airui said.
In the future, HAOMA will draw an area in the landing city to indicate whether the city navigation function can be activated. The range of the area may be determined based on the amount of data and road complexity in the area.
Advancements in Core Algorithms
The advancement of the core algorithm is the basis for HAOMA to set the goal of landing in 100 cities by 2024.
At the recent AI DAY, HAOMA released five models of MANA, including visual self-supervision, multi-modal mutual supervision, 3D reconstruction, dynamic environment, and human driving self-supervision cognition.
Among them, the multi-modal mutual supervision model and the dynamic environment model are large models used in the vehicle end to improve the HAOMA MANA perception framework.
The multi-modal mutual supervision model is similar to Tesla’s released occupancy network model last year in terms of effect. Its core is to use visual data to model the surrounding space in real time, constructing the spatial structure information that only has length, width, and height, but does not have semantic information such as “bus station”, “water horse”, “passenger car”, and “pedestrian”.
Therefore, it can directly avoid the occupied position on the road and plan the drivable space.
Moreover, since there is no need to determine what is perceived, the real-time computing power demand on the vehicle end can be greatly reduced, even the precision requirement of the camera, which can be landed on a low-cost perception and computing platform.
However, on the other hand, vision can only provide 2D information, and adding depth and time information requires high requirements for data quantity and model tuning.
In addition, there are certain differences in the implementation methods and effects between the multi-modal mutual supervision model of HAOMA and the occupancy network of Tesla.”
Tesla fully relies on pure vision, while incorporating laser radar information that directly acquires 3D information to supervise the vision perception results.
Archer said, "We hope to achieve the same effect as laser radar using only pure vision in the future. Although there is no laser radar on this car, the result obtained is equivalent to having a high-speed laser radar installed."
The dynamic environment big model is similar to Tesla's lane model, which constructs a real-time road topology structure through semantic understanding of the "seen" roads.
Therefore, the model can, like an experienced driver familiar with the road conditions, plan and drive in real-time based on the observed actual road conditions after knowing the approximate path and direction in advance, freeing itself entirely from the constraints of high-precision maps.
After sufficient validation, Archer hopes that the dynamic environment model can correct the map information as a credible party in the future. "In the computer field, when you have sufficient data, you will find that letting the model make choices may be more suitable than summarizing the rules yourself."
As of now, the accuracy rate for advanced topology inference of 85% of intersections achieved 95% in Baoding and Beijing.
Currently, the dynamic environment big model is still being trained in the cloud and has not yet landed on the car end.
## Massive investment in infrastructure
To rely on multimodal mutual supervision and the dynamic environment big model to reduce reliance on laser radar and high-precision maps requires massive infrastructure investment.
The big model refers to neural network models with parameters reaching 1 billion levels or even higher, which can handle more complex and diversified tasks. However, at the same time, large models require massive data for training, and because of the huge model and parameters, efficient training requires huge computing power and speed.
Therefore, Tesla not only further strengthens the automatic closed-loop of its data annotation but also builds its large-scale smart computing center called DOJO.
Similarly, the low-cost, universal-oriented Waymo has established a similar infrastructure system.
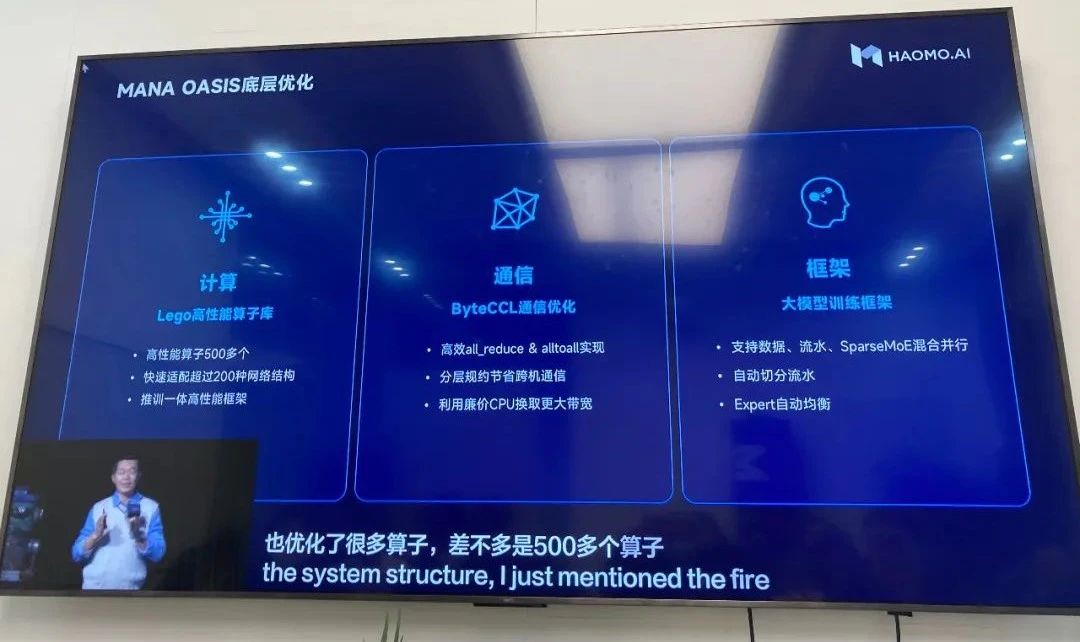
markdown
Manbang’s smart computing center, “Mana Oasis,” has been completed, achieving 67 billion billion floating point operations per second, 2T storage bandwidth per second, and 800G communication bandwidth per second. It can handle the demands of large-scale model training for data volume, throughput, and computing efficiency, and has a latency of less than 500 microseconds for random read and write of billions of small files.
XPeng Motors’ Feiyang computing center has achieved 60 billion billion floating point operations per second. The peak performance of the Sunway TaihuLight supercomputer is 12.5 billion billion operations per second and the sustained performance is 9.3 billion billion operations per second.
With a floating point computing power of 67 billion billion times, the cost is about RMB 1 billion (based on a USD-RMB exchange rate of 6.8) when calculated at a price of $32,000 per NVIDIA A100 GPU.
Manbang had previously cooperated with Alibaba Cloud in building the computing center, but the decision to build its own center shows its determination. In addition to building its own computing center, Manbang has also improved its data processing capabilities by using learned models.
The visual self-supervised large-scale model achieves one-time 4D annotation that includes time continuity across frames, and provides improved annotation for single-frame data that was previously unannotated, reducing annotation costs by 98\%.
The 3D reconstruction large-scale model can simulate and reconstruct real scenes, and obtain a large number of corner cases.
The human-driven self-supervised cognitive large-scale model is similar to a shadow mode, training a more human-like driving posture and strategy through feedback from human drivers taking over control.
According to Zhang Kai, in addition to customers within the Great Wall system, Manbang has also reached cooperation intentions with customers from other brands. Currently, Manbang’s simulation work covers more than 70\% of the research and development process, and research and development efficiency has increased by 8 times compared to two years ago. In terms of engineering, a first-pass yield rate of 100\% is achievable for smart driving products.
“`
This article is a translation by ChatGPT of a Chinese report from 42HOW. If you have any questions about it, please email bd@42how.com.