Wu Enda’s autopilot start-up Drive.ai to launch autopilot test operation in Frisco, Texas, in July this year
Wu Enda promoted a piece of news on all his social media accounts today: Drive.ai, an autopilot start-up where he serves as a director, will launch an autopilot car test operation in Frisco, Texas, USA in July.
Here is the specific execution plan:
Phase 1: The autopilot car test operation will be deployed in Texas in July. There are several key points:
- Equipped with safety drivers who can take emergency control in case of unexpected road conditions;
- Within a limited area, there will be on-demand, multipoint combinations, which is a primary big data network scheduling;
- Support remote control of the car, and the system will also learn from human operations in the background.
Phase 2: When the technology level is safe enough, remove the safety driver and assign “chaperones”. For those who are not familiar with the term, it means that there will still be safety drivers, but they will not sit in the driver’s seat, but in the passenger seat and are responsible for assisting other passengers and monitoring the driving system.
Phase 3: Completely remove the safety drivers, and the remote control in case of emergency situations handed over to the background.
The emphasis of the third phase is that Google Waymo is currently testing a true unmanned vehicle that does not require a safety driver in Arizona, and it also supports remote control in case of emergency situations. Drive.ai may be following this idea.
Wu Enda said that Drive.ai has optimized the remote control system to have good robustness when encountering network delays or temporary signal interruptions. For example, if data is lost or delayed by 100 milliseconds, the system can respond in real-time.
In other words, if Uber also has similar control mechanism, even if the safety driver fails, the background control can also avoid tragedy?
From this perspective, Drive.ai’s consideration of the operation and interaction of the system is still relatively comprehensive, which is one of the details in Drive.ai‘s product design.
Below are the autopilot cars that will be launched in July, based on Nissan NV 200 refitting, and the hardware sensors include 10 cameras, 2 radars, and 4 16-line lidars.
# Based on the modification of the Nissan NV200, we are seeing an autonomous driving car for the first time, which was actually hinted at by the co-founder of Drive.ai, Wang Tao, as early as the beginning of last year. According to Wang, they saw the potential for the NV200’s dual-purpose for both passengers and cargo, as well as the potential for electrification modifications. The Nissan NV200 comes in both fuel-powered and new energy versions, but uses the same protocol, making it very friendly for modification and control.
In addition, you may have noticed that the car is equipped with screens on all sides, as well as the bright orange paint job, both of which are intended to enhance safety. The screens are used for human-to-car interaction and interaction with pedestrians, cyclists, and other cars.
Of course, this was not inspired by the lesson learned from the Uber accident. Drive.ai had actually showcased a prototype of their car in early 2018, emphasizing the importance of external interactions.
Now, let’s talk about the interesting technology behind it.
Drive.ai became famous in Silicon Valley’s crowded field with the video of their autonomous car driving in rainy road conditions, which was released before Tesla and Waymo in February 2018. The four-minute video showed the driver not touching the steering wheel or making any interventions throughout the entire journey.
They were able to achieve this video presentation effect in a very short period of time, and the deep learning-first technology strategy behind it showed enormous potential.
Drive.ai CEO Sameep Tandon succinctly described Drive.ai’s technological advantage: while most companies use deep learning technology as a tool for one aspect of autonomous driving, Drive.ai applies deep learning to the entire process of autonomous driving.
Specifically, Drive.ai’s approach is based on deep learning network models for decision-making routes using non-regularized learning. This approach has the following three advantages:Generalization of Scenarios: Traditional rule learning is difficult to reliably deal with new and rare situations, while non-rule learning’s generalization ability allows it to better understand data and solve such long-tail problems in real-time.
Complex Decision-Making: Non-rule learning supported by a large number of examples has advantages in complex decision-making, as demonstrated by deep learning programs such as AlphaGo. For a long time in the future, there will be both autonomous and manual driving vehicles on the roads, and the complex decision-making (whether to overtake, change lanes, etc.) required for autonomous driving is similar to that of Go-playing – making next moves based on the opponent’s actions and reasonably obtaining right of way.
Hardware Requirements: Using non-rule learning instead of a ready-made knowledge base for decision-making requires relatively low efficiency from the computing chip, and core data processing can be completed on in-vehicle devices with computing power equivalent to a personal computer.
However, deep learning models of non-rule learning also have some problems. For example, the algorithm is not interpretable: in the “end-to-end” training, there are opaque areas in policy planning, and the principles and mechanisms are not clear (“black box”). But companies must ensure that the decision-making behavior of autonomous vehicles is transparent and traceable, so as to make improvements in case of accidents. If it is opaque, there will be no room for improvement. In addition, transparency and traceability are also critical for determining the party responsible for accidents.
In an interview last year, when asked about this issue, Wang Tao’s response was that Drive.ai would adopt the mainstream generative adversarial networks (GANs) in academia, and split the entire end-to-end system into different parts for validation and testing on standardized test machines covering the vast majority of scenarios.
Why adopt a deep learning priority strategy? There are a total of 8 co-founders of Drive.ai, 6 of whom were students of Andrew Ng during his time at the Stanford Artificial Intelligence Laboratory, including CEO Sameep Tandon. More importantly, the president of Drive.ai is Andrew Ng’s wife, Carol Reiley, who is the driving force behind Andrew Ng’s endorsement of Drive.ai as a director.
There is no need to say much about Andrew Ng’s appeal and influence, as Baidu Baike accurately describes him as one of the most authoritative scholars in the field of artificial intelligence and machine learning internationally.
Before this, Drive.ai has partnered with Lyft to launch a trial operation of self-driving taxis in the Bay Area and has received investment from Southeast Asia’s ride-hailing giant Grab, preparing to test self-driving cars in Singapore.
The boom of self-driving startups that began in early 2016 has finally come to the time to turn in their work. The startups in the bubble period are similar, but their operations during the winter period have their own misfortunes. Some have already been acquired, but Drive.ai’s story seems to continue.
-
Exclusive Interview | He Tao: The story behind the world’s first commercialized autonomous sweeping robot
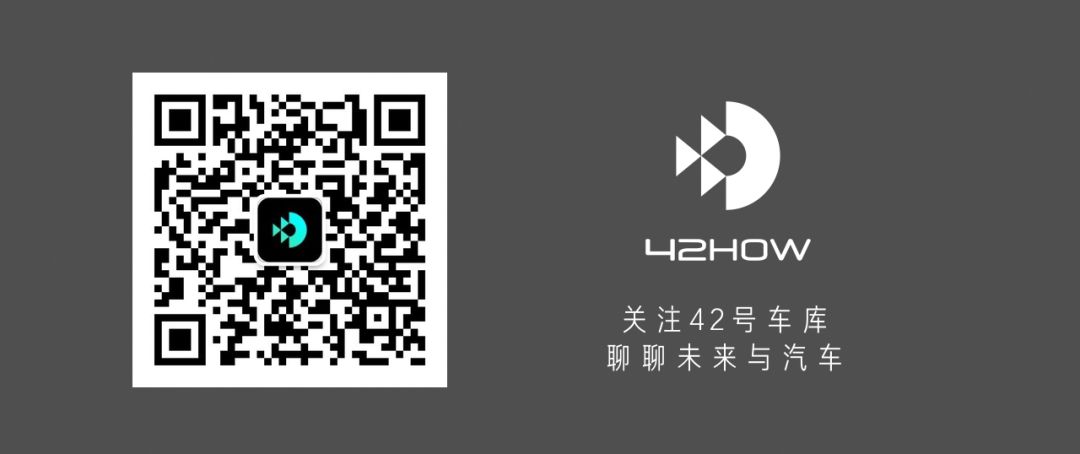
This article is a translation by ChatGPT of a Chinese report from 42HOW. If you have any questions about it, please email bd@42how.com.